Red Dot Payment DEEPER DATA EXPLORATION AND ANALYSIS
Initial Data Exploration and Analysis | Deeper Data Exploration and Analysis | Setting Benchmarks for Merchant Performance | Limitations | Implications |
---|
After establishing a positive correlation between the number of transactions and the number of approved transactions, we wanted to discover possible correlation of any other independent variables with the number of approved transactions.
The following key merchants were analyzed from each bin: (i) Best star merchant, (ii) Worst laggard merchant, (iii) Merchant with highest number of transactions.
Firstly, to firm binary variables, we recoded all the different rejected transaction reason code descriptions into 'Rejected’, and approved transactions remained as ‘Approved’.
To illustrate the general trend, we will showcase the logistic fit results for the merchant with the highest transaction volume in bin 4 (Merchant M1).
LOGISTIC FIT
In this section, we wanted to find out if variables such as monetary value and time of transaction would have a correlation with the approved-to-rejected transaction ratio for each merchant. In this case, we refer time to ‘Month’ of the Transaction, ‘Day’ of the Transaction and ‘Time of the Day’ of the Transaction.
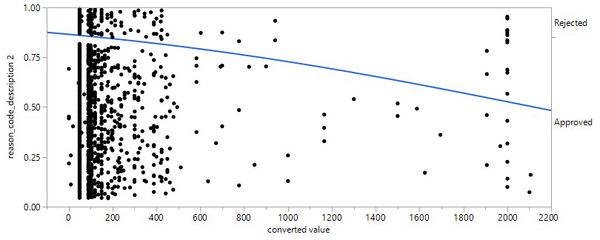
Figure 13: Logistic fit graph of approved/rejected transactions against monetary value for M1
Figure 13 showcases the logistic fit analysis performed on M1, by plotting reason code description against monetary value. A negative correlation is observed. This trend applies to all other merchants, with one exception, as seen in Figure 14.
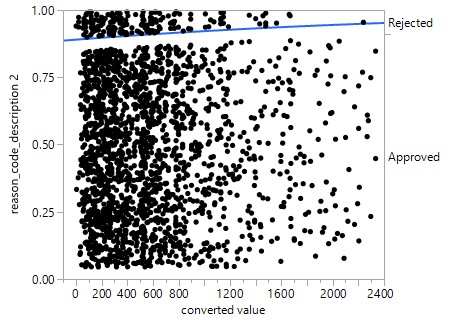
Figure 14: Logistic fit graph of approved/rejected transaction against monetary value for star merchant in Bin 4
Figures 15a-15c showcase the logistic fit analysis performed on M1, by plotting reason code description against time of transaction. However, we could not observe any discernable patterns between approved-to-rejected transaction ratio and time. This demonstrates that there is no significant relationship between the two variables, and it applies to all other key merchants.
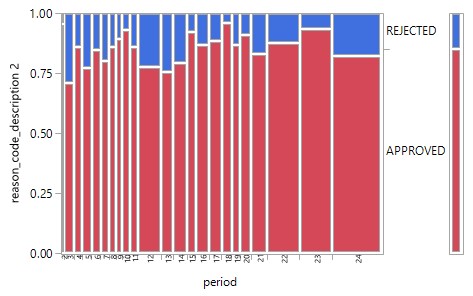
Figure 15a: Logistic fit graph of approved/rejected transaction against month of transaction for M1
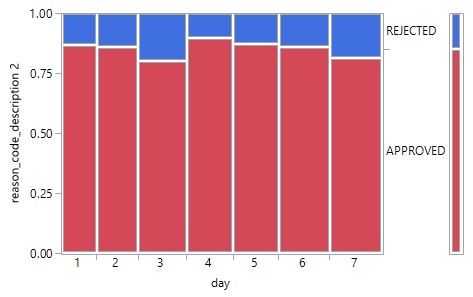
Figure 15b: Logistic fit graph of approved/rejected transaction against day of transaction for M1
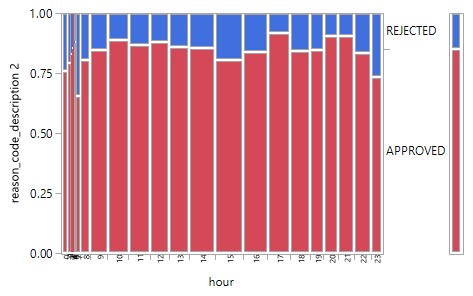
Figure 15c: Logistic fit graph of approved/rejected transaction against hour of transaction for M1
Thus, we conclude that only monetary value of transaction has a negative correlation with number of approved transactions.
DECISION TREE
The monetary value of transaction was the only independent variable observed to have negative correlation with the number of approved transactions. Going further, we used decision trees to analyze and identify the cut-off monetary value of a transaction that leads to lower approved-to-rejected transaction ratio.
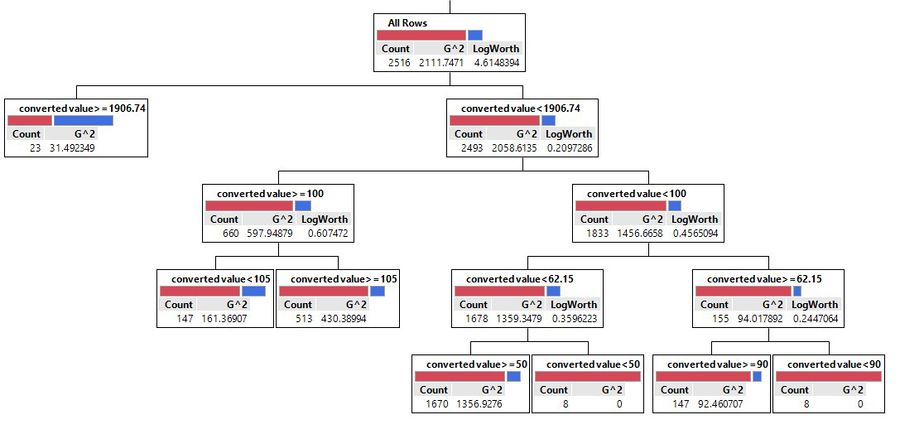
Figure 16: Decision tree on monetary value for M1
Figure 16 shows us that for monetary value of transactions above SGD1,906.74, there is a lower approved-to-rejected transaction ratio as compared to the transaction with monetary value below SGD1,906.74 (Red – Approved transactions; Blue – Rejected transactions).
The table below summarizes the insights observed from logistic fit and decision tree analysis of each bin.
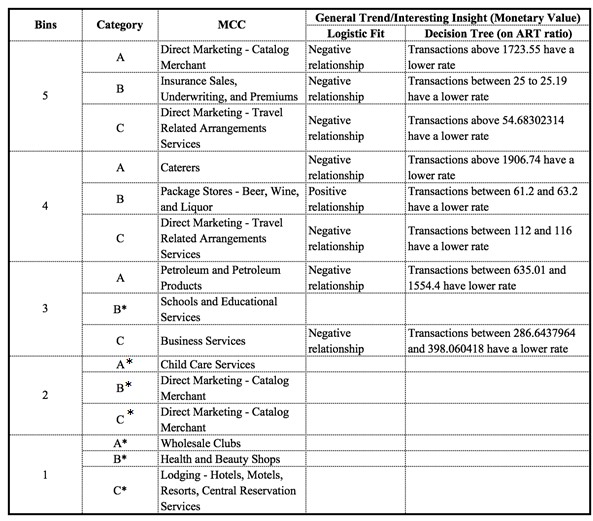
Figure 17: Summary table for results from logistic fit and decision tree analysis A - merchant with the highest transaction volume, B - best star merchant, C - worst laggard merchant