Sofia City's Air Pollution Problem Analysis
Viz & Insights
Spatio-temporal Analysis of Official Air Quality [Task 1]
The Daily / Hourly Trends. View the interactive Tableau design here: [1]
Patterns |
Visualization |
1. Setting the scene – General Overview of the data readings
The pollution recordings were broken down into monthly series and it was observed that the data between Feb 17 to Oct 17 is missing. To prevent the missing data from skewing the rest of the analysis, we exclude all readings taken in 2017.
It is also essential to note that the data has been transformed into two data sets based on time frame, namely daily and hourly.
The hourly data has been recomputed into daily, by averaging out the hourly readings, and added to the daily data set. This allows for clearer investigation by first looking at the daily observations, before delving and zooming into the hourly data set.
.
|
|
2.Seasonality
When aggregating the average data taken across the official stations, it is clear that the average readings for months in Dec and January are above the stipulated safety level by EFA for PM10. As this is an overall outlook, we look zoom into respective years across the respective stations to see if there are any interesting pointers worth investigating.
The trend appears to be relatively consistent across the years. Thus when looking at the meteorological parameters we will see if there are similar patterns, proportionally or inversely proportionally, that may explain then pollution trend.
|
|
3. In-depth Analysis (Daily Reading)
Station - BG0040A: PM10 readings start to pike in Nov and drop in Jan. Minimal breach of safely level between Feb to Oct. This trend is consistent across the years.
Station - BG0050A: PM10 readings start to pike in Nov and drop in Jan. Minimal breach of safely level between Feb to Oct. This trend is consistent across the years.
Station - BG0052A: PM10 readings start to pike in Nov and drop in Jan. But readings Dec and Jan are exceptionally high. in Minimal breach of safely level between Feb to Oct. This trend is consistent across the years.
Station - BG0054A: The station has ceased to take pollution readings since Oct 2015. Station has not been taking records for the past 3 years.
Station - BG0073A: PM10 readings start to pike in Oct and drop in Feb. While the severity of pollution seems to dip since 2016, the pollution in the months of Dec and Jan remains dreadful.
Station - BG0079A: Station began its operations in 2018.
|
|
4. In-depth Analysis (Hourly Readings)
The Hourly Readings were taken from 2016. However the not all the readings were taken consistently across the stations. As we have also excluded data set from 2017 due ti missing readings, we will be investigating on the hourly readings across stations from the Jan 2018 onwards.
Jan Readings was high as expected.
Anamoly Servere pollution readings observed on :
1) wee hours of 17 & 18 Feb (stations BG0050A, BG0073A, BG0079A)
2) wee hours of 18 Mar (stations BG0050A, BG0052A, BG0073A, BG0079A. Data Missing for BG0040A.)
3) morning for the month of Apr at station BG0040A.
4) no significant findings for the rest of 2018.
|
|
5. Problems
The presence of these pockets of missing data in both daily and hourly readings, as well as absence of 9 months worth readings in 2017 prohibits the thorough analysis of the pollution situation at Sofia City. The absence of a comprehensive data will diminish the effectiveness of environmentalists and authorities from crafting effective policies to tackle the problem as they may not be able to sieve out the root cause. Moreover, the data that was available was only on PM10 and there were no data made available on PM2.5. It is important to analyse the presence of PM2.5 as it brings about worsen and detrimental situation to the people of Sofia City.
Though the station types are differentiated between background and traffic, there are no visible differences when analysing between these two types of stations. For traffic data, there are no higher readings recorded during the usual working peak hours nor weekdays versus weekends.
However in the case of Sofia, the emergence of AirBG, a citizens' initiative to address the awful air pollution, has vastly boosted efforts to garner air pollution readings as well as meteorological data. We will now investigate the Citizen's reading on the next part. |
Spatio-temporal Analysis of Citizen Science Air Quality Measurements [Task 2]
The Hourly Trends (2017) View the interactive Tableau design here: [2]
The Hourly Trends (2018) View the interactive Tableau design here: [3]
Patterns |
Visualization |
---|
1.Data Collection
The data collected spans from September 17 to April 2018. The data in 2017 was collected by 386 unique households and in 2018, collected by 1096 unique households. The type of data collected was hourly readings of P1 (PM10) and P2 (PM2.5) respectively. P1 and P2 was identified as the respective PM based on the range of data collected. Other geographical data collected at Temperature, Pressure and Humidity. Latitude and Longtitude of the location of these citizens meaursement devices were collected as well. |
|
2. Analysis
To ensure the data reminds relevant, there was an initial plan to extract the data that were located in Sofia City. However the Tableau was unable to handle the demand and constantly broke down while trying to sieve out identified data. Thus after 3 crashes, decision was made to move on.
Without the presence of Lat and Long data, we were unable to eliminate readings that were taken beyond Sofia City. Based on the initial geographical image was loaded before the crash, we can see that more than 50% of the citizens' measurement devices were located in Sofia City. Moving on, an analysis was done linking the pollution level, to temperature, pressure and humidity. It is also critical to note that there were missing data from the devices, thus results generated are not the most accurate.
|
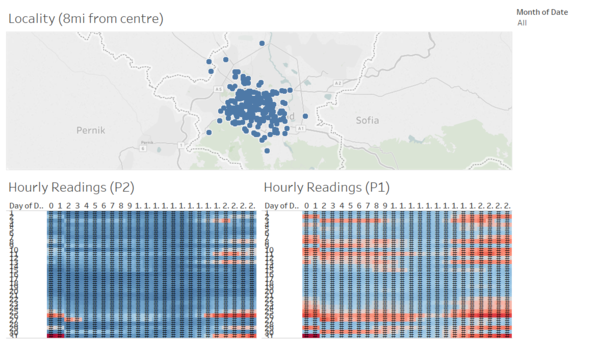 |
3.Results
Looking at the results the readings of P1 and P2 are strongly correlated. The centre attributed to P1 and P2 are 50 μg/m3 and 40 μg/m3 respective based on recommended figures by EU. The temperature readings are strongly inversely related to the pollution data. This is identical to the results found in task 1. There were no such observations for pressure against the pollution data.
The pressure readings were consistent during each day, regardless of the time of the day. As for Humidity, the readings were lower during daylight hours for the last 4 months of the year, but more consistent throughout the day in the first 4 months of the year. It was important to note that there were more devices taking readings in 2018 and the inability to sieve out the errant ones could be the factor behind different observations made on humidity in 2017 and 2018 |
|
Integratio of Data Set [Task 3]
Meterological Data View the interactive Tableau design here: [4]
We take the anomalies observed from Task 1 and Task 2 for further investigation.
Anomalies |
Visualization |
1. In task 1, we noticed that the PM10 pollution readings peaks in the months of Dec and January. Upon investigating the seasonal trends of the various meteorological data, we notice that the temperature and dew point temperature are low during these periods. This pattern is consistent through the years of record. This phenomenon is explainable by the scientific theory where "hot air rises, cool air sinks". General air molecules contracts during winter and this results in the concentrated as they are heavier and is now trapped in the valley.
The situation is exacerbated during the months of severe pollution when the temperature hovers around 0 degree and many households than to switch on their heating appliances. As the nice warm air from the houses leaks out, the cold and now more polluted air leaks in. The more openings the house and larger difference between indoor and outdoor temperature, the tendency for indoor pollution level to rise. (Potentially higher pollution readings indoor than outdoor)
|
|
2.There are no other anomalies found associated between the meteorological readings and pollution data. Given the geographical location, in a valley and as a basin, there is not much variation to the climate. Visibility range is the effect of the pollution situation. There appear to be no other weather-related causations to the pollution situation in Sofia City. 3. When looking at the geographical data, we realised all the measuring station, official or civilian, are the location within the city level. None were placed at a higher altitude to provide a different altitude outlook. Thus we are unable to provide further insights on the comparison between topography and meteorological characteristic.
There was an exceptionally high rainfall in November 2016 but there was no difference to the pollution data recorded.
|
|
3. There are no other anomolies found associated between the meterological readings and pollution data. Given the geographcial location, in a valley and as a basin, there are not much variation to the climate. Visibility range is the effect from the pollution situation. There appears to be no other weather-related causations to the pollution situation in Sofia City.
|