ANLY482 AY1516 G1 Team Skulptors - Outbound EDA
![]() |
![]() |
![]() |
![]() |
![]() |
![]() |
Data Cleaning | Inbound EDA | Outbound EDA |

For the outbound data, there are limitations on the analysis on the pick rate of the warehouse stock as for our inbound, we are largely concerned with the pallets inbound (Warehouse stores items in pallets) but for outbound, we are mostly concerned with quantity. This is because not all warehouse outbound movements are large quantities – e.g. a company might only need 50 syringes instead of a carton to top up their medical kit.
From the picking rate, we can see an average of 500 quantity picked for each row of outbound movement. The standard error of the mean is relatively low as well (23.36), which shows that the bound on the mean movement is relatively small, depicting consistency in the outbound quantity. Our group noticed a large movement of 1440000 quantity. We believe that this outlier is probably due to an error in entry on the company’s part, since an outbound quantity of 1440000 is extremely high. If this is not an error, a possible deduction could be that the item is tiny such as an alcohol swab.
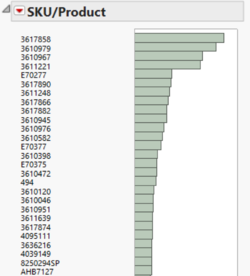
For outbound, popularity of SKUs/products is very important as they influence the inbound rates of the product – if a certain product is fast moving, it shows that the product is popular, therefore more inbounds are required to satisfy the demand. Popularity of the product can in turn predict the movement of the product, which is information useful to the warehouse manager. He or she can then allocate spaces accordingly for the popular or unpopular products. We sorted the outbound frequency of the SKUs/products in descending order. From the distribution analysis above, we can see that the top 4 products account for much difference compared to the outbound movements for other products. There is a possibility that the first 3 digits (361) depicts a type of good or a certain attribute of the product, which results in most products that start with product code 361 to be generally more popular. In the list, the top 10 SKUs have are mostly products with product codes that starts with 361.
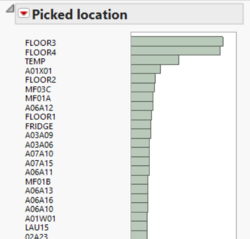
Similar to the inbound data, the top picking location – where the stock is being picked up from, revolves around the floor or the temporary location. However, one point to note would be the most popular location. In the inbound, majority of the product stock keeping units are stored in the TEMP location, which our group believes is the temporary location. But in this graph, instead of TEMP being the most popular location for picking, it is the floor areas FLOOR3 and FLOOR4 instead. A distinct problem is shown here - If most of the inbounds are stored at the TEMP location, but most of the outbound is picked from the FLOOR locations, wouldn’t there be a problem of overloading over at the TEMP location? We foresee this issue to be a problematic, which requires further investigation. As for the other locations, they are generally quite even in terms of picking rate, but our group feels that it can be utilized better, considering most of their picking location revolves around the floor and the temp locations.
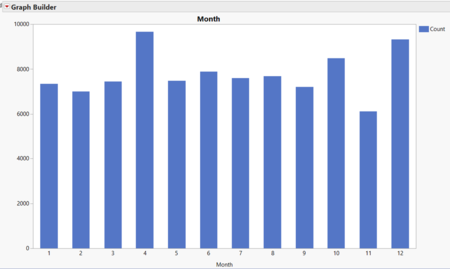
In this graph, our group looks at the distribution of the outbound on a monthly basis. There is a general consistency in the outbound, with a minimum of 6122 in November 2015 (Month 11) and a maximum of 9654 in the April 2015 (Month 4). However, this graph is limited in a sense it only shows outbound frequency by monthly basis. This graph does not show the volume of outbound – i.e. a month might have very little outbound, but each outbound is a large outbound of more than 1 million pieces. This graph is still useful as it shows a general overview. This is also because based on our previous findings, we notice the pick rate mean and bound is relatively consistent, so each outbound is roughly identical in outbound size.
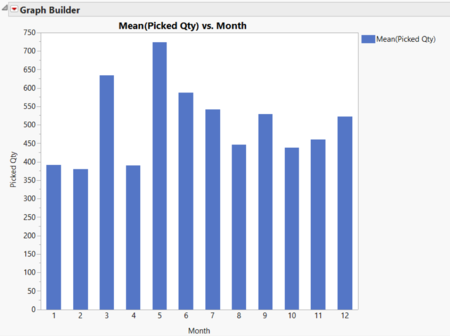
To investigate on the volume scenario, we plotted a bar graph with y-axis consisting of picked quantity versus the x-axis time dimension, month. Here we can see the average picked quantity of outbound in each month, which shows much more variation as compared to the previous graph. These two graphs can be used hand in hand to show volume of outbound. As mentioned before, the outbound is limited due to the use of measurement – pieces versus pallets. The number of pallets consumed is not shown in the outbound data, thus we are restricted to only pieces. From a warehouse point of view, a unit measure of different products can be vastly different in space occupation – a syringe and an alcohol swab is both 1 unit of measure in piece, but an alcohol swab is definitely much smaller compared to a syringe.
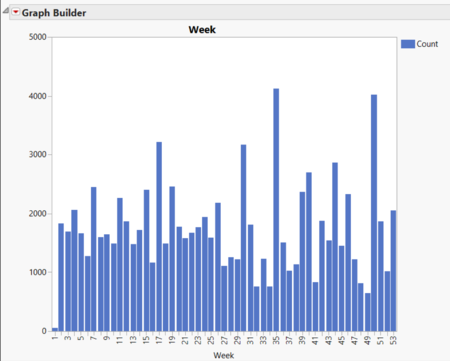
This graph further slices the time dimension to a weekly level analysis. In this graph, we can see generally in each week, there is at least one outbound. However, there is shockingly vast differences in outbound rates for each week in the 2015 working year. It can go as low as 61 times in the first week to 4060 times in week 35. This is cost-heavy and taxing on the warehouse employees as with such vast differences in outbound rates, the warehouse employees have to cater to this fluctuations which are relatively volatile. This can be seen from the graph above, where the outbound rate jumps around at random from high to low and vice-versa. Ideally, with proper planning, the outbound rates can be relatively consistent and controlled. There are two scenarios that can help warehouse employees:
1. Periodic outbound: Outbound rates are pushed seasonally so warehouse employees will know when to account for high and low pick rate periods
2. Consistent outbound: If outbound rate is kept relatively constant and within control, it is much easier for the warehouse to prepare for these outbound
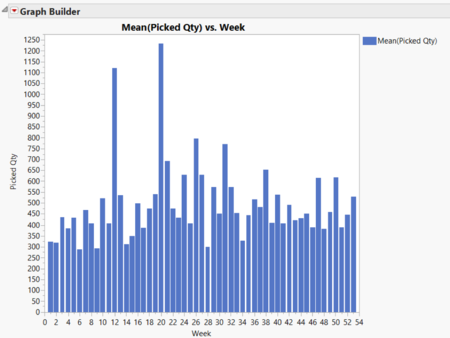
Similar to before, we include the picked quantity in the y-axis versus the x-axis time dimension, week. The variations are similar to the pick rate count in the previous graphs, but in terms of average picked quantity, they are still more controlled than before, with the exceptions week 12 and week 20. These two weeks peaked the mean picked quantity, exceeding a thousand quantity picked in a given week. This graph can be used hand in hand with the previous graph to show the volume of outbound rate on a weekly basis.
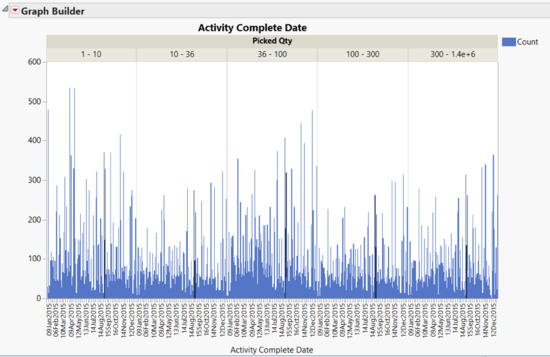
In this graph, we wanted to see the dates where each group of picked quantity is commonly found in. We segregated the picking quantity into 5 categories, 1-10, 10-36, 36-100, 100-300, and more than 300. The reason the groups are not uniform in picking quantity size is because these picking sizes are packaged in such a way – anything less than 10 pieces is taken loosely, 10-36 will be in a box, 100-300 in a carton, anything more than 300 needs to be in a pallet etc. These segmentations will give a better representation of the frequency of different quantity outbound. Based on the graph, we can see that all categories have a healthy consistency, with 1-10 picked quantity ranging slightly more. A peculiar fact that can be helpful to the company is the Activity Complete Date (Day where the goods are picked). Other than the 1-10 category, all other categories are more common in the later portion of the year.