Difference between revisions of "ANLY482 AY1516 G1 Team Skulptors - Inbound EDA"
(Created page with "<!--Main Navigation--> <center> 160px|link=ANLY482 AY1516 G1 Team Skulptors {|style="background-color:#ffffff; color:#000000; width="100%" cellspa...") |
|||
Line 32: | Line 32: | ||
<br/> | <br/> | ||
[[Image:Skulptors-PutawayLocation.png|left|220px|link=]] | [[Image:Skulptors-PutawayLocation.png|left|220px|link=]] | ||
+ | <br/><br/><br/> | ||
+ | Most of the inbounds putaway location is TEMP or FLOORS. This indicates a detrimental issue of overloading, whereby the warehouses ran out of space to store the goods. There can be another possibility, where the storehouse is not properly utilized, and thus temporary and floor areas are used predominantly. | ||
+ | <br/><br/><br/><br/><br/><br/><br/> | ||
+ | |||
+ | <div align="left"> | ||
+ | <div style="background: #d9d9d9; padding: 12px; font-family: Impact; font-size: 18px; font-weight: bold; line-height: 1em; text-indent: 15px; border-left: #595959 solid 32px;"><font color="black">Pallet Analysis</font></div> | ||
+ | <br/> | ||
+ | [[Image:Skulptors-Pallet.png|left|220px|link=]] | ||
+ | <br/><br/><br/> | ||
+ | As a warehouse, quantity of products might not be as prevalent a contributing factor as compared to the number of pallets. The warehouse itself contains shelving location for such pallets to be stored in. Each shelf is able to store 1-3 pallets. However, as seen from the distribution analysis, the number of pallets vary from 1 to a whopping 669 pallets (Overloads the whole warehouse). Although these scenarios are not common, it only takes one inbound of 669 pallets to cause a problem of overloading. This is similar to a dog-food distribution, with a heavy positive skew. Based on the right skew, the mean value will be greater than the median value, thus the median would be a better choice for estimation in this scenario. | ||
+ | <br/><br/><br/><br/><br/><br/><br/> | ||
+ | |||
+ | <div align="left"> | ||
+ | <div style="background: #d9d9d9; padding: 12px; font-family: Impact; font-size: 18px; font-weight: bold; line-height: 1em; text-indent: 15px; border-left: #595959 solid 32px;"><font color="black">Pallet By Receipt Date Analysis</font></div> | ||
+ | <br/> | ||
+ | [[Image:Skulptors-PalletByReceipt.png|left|500px|link=]] | ||
<br/><br/><br/><br/> | <br/><br/><br/><br/> | ||
− | + | This graph shows the number of pallets received by date. From this graph, we can deduce that in general, there is a consistent inflow of B.Braun products regardless of dates. However, there are outliers as shown by the representation of 669 pallets. | |
+ | <br/><br/><br/><br/><br/><br/><br/><br/><br/><br/><br/> | ||
+ | |||
+ | <div align="left"> | ||
+ | <div style="background: #d9d9d9; padding: 12px; font-family: Impact; font-size: 18px; font-weight: bold; line-height: 1em; text-indent: 15px; border-left: #595959 solid 32px;"><font color="black">Mean (Number of Pallet) by Month Analysis</font></div> | ||
+ | <br/> | ||
+ | [[Image:Skulptors-PalletPerMth.png|left|450px|link=]] | ||
+ | <br/><br/><br/><br/><br/> | ||
+ | This diagram shows the average number of pallets coming in to the warehouse by month. As shown from the graph, there is generally two peak periods of inbounds, Q2 – April to June, and Q4, the end year period. This information would be useful for warehouse managers to have a brief insight on storage preparation to ensure less temp and floor location usages. | ||
+ | <br/><br/><br/><br/><br/><br/><br/><br/><br/><br/><br/> | ||
+ | |||
+ | <div align="left"> | ||
+ | <div style="background: #d9d9d9; padding: 12px; font-family: Impact; font-size: 18px; font-weight: bold; line-height: 1em; text-indent: 15px; border-left: #595959 solid 32px;"><font color="black">Mean (Number of Pallet) by Week Analysis</font></div> | ||
+ | <br/> | ||
+ | [[Image:Skulptors-PalletPerWeek.png|left|450px|link=]] | ||
+ | <br/><br/><br/> | ||
+ | This graph further slices the time dimension to a weekly level analysis. In this graph, we can see generally in each week, there is at least 1 pallet coming in on average. Week 25 and week 51 are the outliers, with both of these weeks reaching an average of 5 to 6 pallets inbound. The rest of the weeks generally fall between 1 to 4 pallets inbound a week on average. In this graph, we decided to use the bar chart because our group feels the bar graph has a better visibility for 52 weeks compared to the line graph. As such, for months we will display using the line graph while for weeks, bar graphs will be used. | ||
+ | <br/><br/><br/><br/><br/><br/><br/><br/> | ||
+ | |||
+ | <div align="left"> | ||
+ | <div style="background: #d9d9d9; padding: 12px; font-family: Impact; font-size: 18px; font-weight: bold; line-height: 1em; text-indent: 15px; border-left: #595959 solid 32px;"><font color="black">Small Inbounds by Month Analysis</font></div> | ||
+ | <br/> | ||
+ | [[Image:Skulptors-SmallInboundByMth.png|left|450px|link=]] | ||
+ | <br/><br/> | ||
+ | In this graph, we continue from the other diagram where we compare the relationship between number of pallets to the time dimension, month. In the previous graph, we look at all pallets, however, each inbound may be a small or large inbound, with regards to the number of pallets per transaction. Thus, our group decided to group all inbound transactions that involve one or less pallets to be a small inbound. Anything more than 1 pallet per inbound is considered a large inbound. The graph shows the average of small inbounds, with 1 being a 100% of inbounds being small and 0 being 0%. From the graph, we can see the lowest percentage of small inbounds is 88.5%, which shows us that a vast majority of B.Braun inbounds are small – one or less pallets. This portrays the problem of maximizing warehouse utilization, as if inbounds are of high frequency, allocation might be difficult and time-consuming. | ||
<br/><br/><br/><br/><br/> | <br/><br/><br/><br/><br/> | ||
<div align="left"> | <div align="left"> | ||
− | <div style="background: #d9d9d9; padding: 12px; font-family: Impact; font-size: 18px; font-weight: bold; line-height: 1em; text-indent: 15px; border-left: #595959 solid 32px;"><font color="black"> | + | <div style="background: #d9d9d9; padding: 12px; font-family: Impact; font-size: 18px; font-weight: bold; line-height: 1em; text-indent: 15px; border-left: #595959 solid 32px;"><font color="black">Small Inbounds by Week Analysis</font></div> |
<br/> | <br/> | ||
− | + | [[Image:Skulptors-SmallInboundByWeek.png|left|450px|link=]] | |
+ | <br/><br/> | ||
+ | In this graph, we continue from the other diagram where we compare the relationship between number of pallets to the time dimension, month. In the previous graph, we look at all pallets, however, each inbound may be a small or large inbound, with regards to the number of pallets per transaction. Thus, our group decided to group all inbound transactions that involve one or less pallets to be a small inbound. Anything more than 1 pallet per inbound is considered a large inbound. The graph shows the average of small inbounds, with 1 being a 100% of inbounds being small and 0 being 0%. From the graph, we can see the lowest percentage of small inbounds is 88.5%, which shows us that a vast majority of B.Braun inbounds are small – one or less pallets. This portrays the problem of maximizing warehouse utilization, as if inbounds are of high frequency, allocation might be difficult and time-consuming. | ||
+ | <br/><br/><br/><br/><br/> | ||
− | + | <div align="left"> | |
+ | <div style="background: #d9d9d9; padding: 12px; font-family: Impact; font-size: 18px; font-weight: bold; line-height: 1em; text-indent: 15px; border-left: #595959 solid 32px;"><font color="black">Large Inbounds by Month Analysis</font></div> | ||
+ | <br/> | ||
+ | [[Image:Skulptors-BigInboundByMth.png|left|450px|link=]] | ||
+ | <br/><br/><br/> | ||
+ | Moving on to the large inbounds, we notice the vice-versa of trends as compared to the small inbounds. Large inbounds do not take up more than 11.5% of the inbounds per month. However, these inbounds can go as high as 500+ pallets, which is much harder to control and maintain as compared to small inbounds. Ideally, the large inbounds should be kept as low as possible as too many of these large inbounds can lead to overloading of the warehouses. | ||
+ | <br/><br/><br/><br/><br/><br/><br/><br/><br/> | ||
− | + | <div align="left"> | |
+ | <div style="background: #d9d9d9; padding: 12px; font-family: Impact; font-size: 18px; font-weight: bold; line-height: 1em; text-indent: 15px; border-left: #595959 solid 32px;"><font color="black">Large Inbounds by Week Analysis</font></div> | ||
+ | <br/> | ||
+ | [[Image:Skulptors-BigInboundByWeek.png|left|450px|link=]] | ||
+ | <br/><br/><br/> | ||
+ | Similar to before, we slice the time dimension to weeks, to see whether the same consistency is valid for the weekly basis. As compared to the small inbounds, there is much less consistency when it comes to large inbounds. Variations range from close to 0 to almost a quarter of the inbounds for a given week. This makes it harder for the warehouses to prepare for large inbounds. In week 38, the percentage of large inbounds went to 23%, an outlier compared to the other weeks. The usual range of inbounds varies from 1% to 16%. From this graph, it is safe to say at least 1% of the inbounds would be large inbounds. | ||
+ | <br/><br/><br/><br/><br/><br/><br/> | ||
+ | <div align="left"> | ||
+ | <div style="background: #d9d9d9; padding: 12px; font-family: Impact; font-size: 18px; font-weight: bold; line-height: 1em; text-indent: 15px; border-left: #595959 solid 32px;"><font color="black">Box Plot for Number of Pallet Analysis</font></div> | ||
+ | <br/> | ||
+ | [[Image:Skulptors-PalletBoxPlot.png|left|450px|link=]] | ||
+ | <br/><br/><br/><br/> | ||
+ | The box plot of the number of pallets for inbound is shown above. As show, the deviation analysis shows that much of the pallets lie close to zero. This is because most of the inbounds come in as one pallet or a half filled pallet. This can be seen from the diagram, where the interquartile range lies entirely near to zero (The inter-quartile range is so small it is closely ranged to zero) | ||
+ | <br/><br/><br/><br/><br/><br/><br/><br/><br/><br/><br/><br/> | ||
+ | |||
+ | <div align="left"> | ||
+ | <div style="background: #d9d9d9; padding: 12px; font-family: Impact; font-size: 18px; font-weight: bold; line-height: 1em; text-indent: 15px; border-left: #595959 solid 32px;"><font color="black">Small and Large Inbound by Ship from Location Analysis</font></div> | ||
+ | <br/> | ||
+ | [[Image:Skulptors-ShippedFrom.png|left|450px|link=]] | ||
+ | <br/><br/><br/><br/> | ||
+ | There are 3 main locations, 224, 407, and 9800000074. For the x-axis, if the number of pallets incoming is less than or equals to 1, the pallet inbound would be “Small”, if not “Large”. From all 3 locations, the number of pallets that inbounds with 1 or less pallets takes majority of the inbounds. Particularly, shipped from location 9800000074 is the most popular. This will be further inspected in the next graph. | ||
+ | <br/><br/><br/><br/><br/><br/><br/><br/><br/><br/> | ||
+ | |||
+ | <div align="left"> | ||
+ | <div style="background: #d9d9d9; padding: 12px; font-family: Impact; font-size: 18px; font-weight: bold; line-height: 1em; text-indent: 15px; border-left: #595959 solid 32px;"><font color="black">Ship from Pie Chart Analysis</font></div> | ||
+ | <br/> | ||
+ | [[Image:Skulptors-ShippedFromPie.png|left|450px|link=]] | ||
+ | <br/><br/><br/><br/> | ||
+ | More than half of the inbound is shipped from location 9800000074. Based on this information, the company can evaluate better allocation of resources to better prepare for inbound shipped from this location. | ||
+ | <br/><br/><br/><br/><br/><br/><br/><br/><br/><br/><br/> | ||
<br/> | <br/> | ||
<!--Content End--> | <!--Content End--> |
Revision as of 22:38, 27 February 2016
![]() |
![]() |
![]() |
![]() |
![]() |
![]() |
Data Cleaning | Inbound EDA | Outbound EDA |
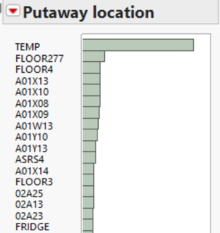
Most of the inbounds putaway location is TEMP or FLOORS. This indicates a detrimental issue of overloading, whereby the warehouses ran out of space to store the goods. There can be another possibility, where the storehouse is not properly utilized, and thus temporary and floor areas are used predominantly.
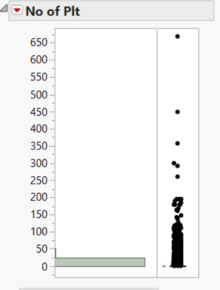
As a warehouse, quantity of products might not be as prevalent a contributing factor as compared to the number of pallets. The warehouse itself contains shelving location for such pallets to be stored in. Each shelf is able to store 1-3 pallets. However, as seen from the distribution analysis, the number of pallets vary from 1 to a whopping 669 pallets (Overloads the whole warehouse). Although these scenarios are not common, it only takes one inbound of 669 pallets to cause a problem of overloading. This is similar to a dog-food distribution, with a heavy positive skew. Based on the right skew, the mean value will be greater than the median value, thus the median would be a better choice for estimation in this scenario.
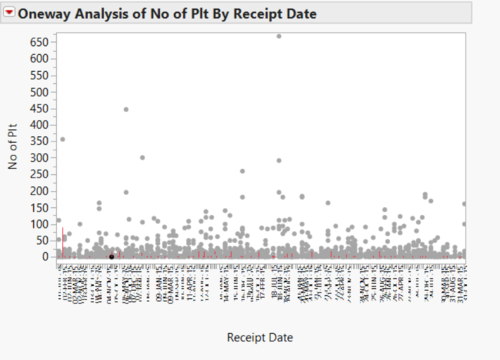
This graph shows the number of pallets received by date. From this graph, we can deduce that in general, there is a consistent inflow of B.Braun products regardless of dates. However, there are outliers as shown by the representation of 669 pallets.
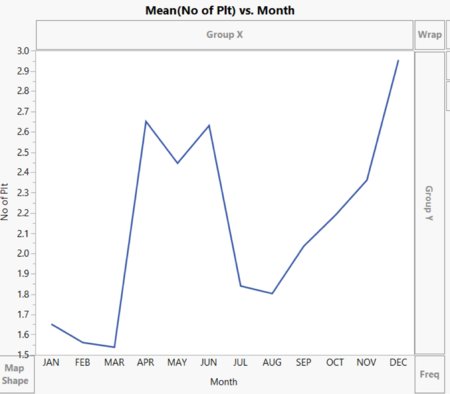
This diagram shows the average number of pallets coming in to the warehouse by month. As shown from the graph, there is generally two peak periods of inbounds, Q2 – April to June, and Q4, the end year period. This information would be useful for warehouse managers to have a brief insight on storage preparation to ensure less temp and floor location usages.
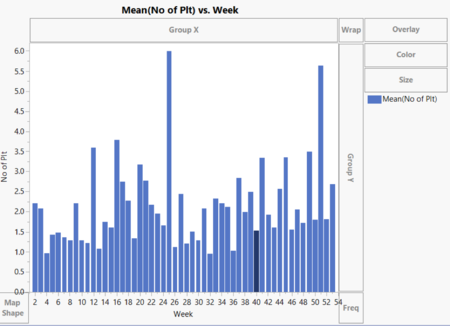
This graph further slices the time dimension to a weekly level analysis. In this graph, we can see generally in each week, there is at least 1 pallet coming in on average. Week 25 and week 51 are the outliers, with both of these weeks reaching an average of 5 to 6 pallets inbound. The rest of the weeks generally fall between 1 to 4 pallets inbound a week on average. In this graph, we decided to use the bar chart because our group feels the bar graph has a better visibility for 52 weeks compared to the line graph. As such, for months we will display using the line graph while for weeks, bar graphs will be used.
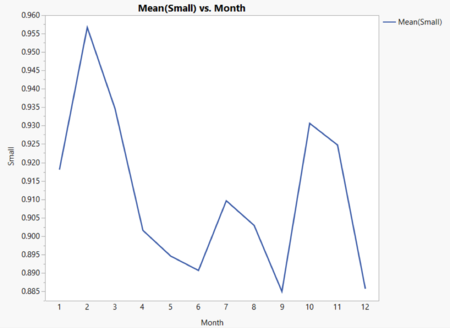
In this graph, we continue from the other diagram where we compare the relationship between number of pallets to the time dimension, month. In the previous graph, we look at all pallets, however, each inbound may be a small or large inbound, with regards to the number of pallets per transaction. Thus, our group decided to group all inbound transactions that involve one or less pallets to be a small inbound. Anything more than 1 pallet per inbound is considered a large inbound. The graph shows the average of small inbounds, with 1 being a 100% of inbounds being small and 0 being 0%. From the graph, we can see the lowest percentage of small inbounds is 88.5%, which shows us that a vast majority of B.Braun inbounds are small – one or less pallets. This portrays the problem of maximizing warehouse utilization, as if inbounds are of high frequency, allocation might be difficult and time-consuming.
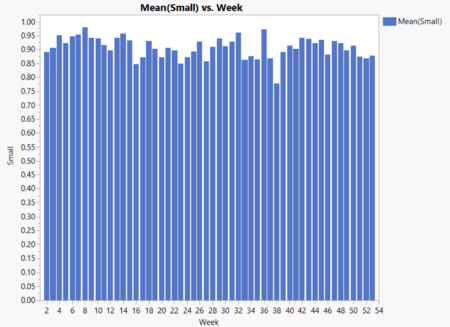
In this graph, we continue from the other diagram where we compare the relationship between number of pallets to the time dimension, month. In the previous graph, we look at all pallets, however, each inbound may be a small or large inbound, with regards to the number of pallets per transaction. Thus, our group decided to group all inbound transactions that involve one or less pallets to be a small inbound. Anything more than 1 pallet per inbound is considered a large inbound. The graph shows the average of small inbounds, with 1 being a 100% of inbounds being small and 0 being 0%. From the graph, we can see the lowest percentage of small inbounds is 88.5%, which shows us that a vast majority of B.Braun inbounds are small – one or less pallets. This portrays the problem of maximizing warehouse utilization, as if inbounds are of high frequency, allocation might be difficult and time-consuming.
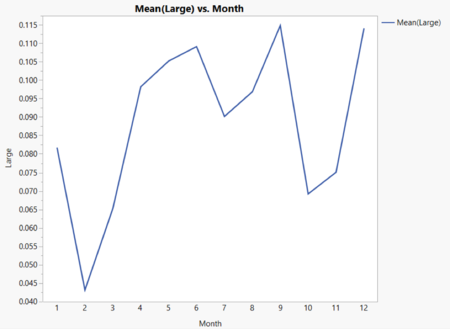
Moving on to the large inbounds, we notice the vice-versa of trends as compared to the small inbounds. Large inbounds do not take up more than 11.5% of the inbounds per month. However, these inbounds can go as high as 500+ pallets, which is much harder to control and maintain as compared to small inbounds. Ideally, the large inbounds should be kept as low as possible as too many of these large inbounds can lead to overloading of the warehouses.
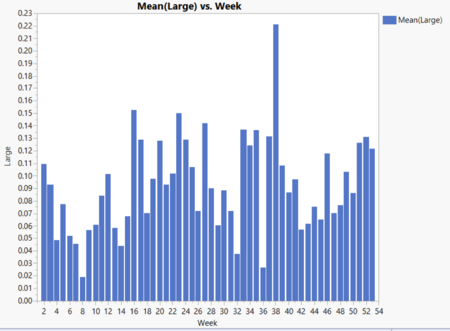
Similar to before, we slice the time dimension to weeks, to see whether the same consistency is valid for the weekly basis. As compared to the small inbounds, there is much less consistency when it comes to large inbounds. Variations range from close to 0 to almost a quarter of the inbounds for a given week. This makes it harder for the warehouses to prepare for large inbounds. In week 38, the percentage of large inbounds went to 23%, an outlier compared to the other weeks. The usual range of inbounds varies from 1% to 16%. From this graph, it is safe to say at least 1% of the inbounds would be large inbounds.
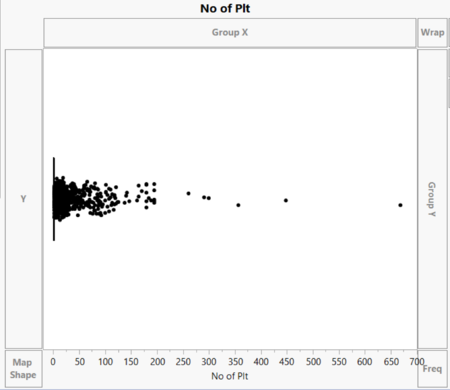
The box plot of the number of pallets for inbound is shown above. As show, the deviation analysis shows that much of the pallets lie close to zero. This is because most of the inbounds come in as one pallet or a half filled pallet. This can be seen from the diagram, where the interquartile range lies entirely near to zero (The inter-quartile range is so small it is closely ranged to zero)
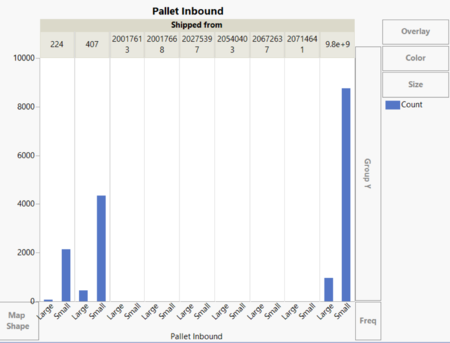
There are 3 main locations, 224, 407, and 9800000074. For the x-axis, if the number of pallets incoming is less than or equals to 1, the pallet inbound would be “Small”, if not “Large”. From all 3 locations, the number of pallets that inbounds with 1 or less pallets takes majority of the inbounds. Particularly, shipped from location 9800000074 is the most popular. This will be further inspected in the next graph.
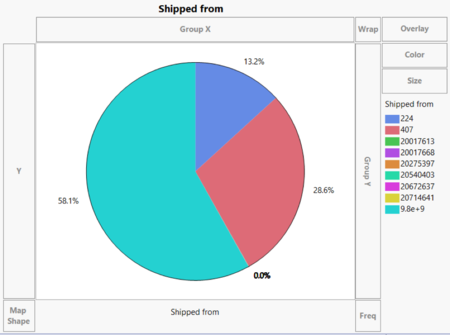
More than half of the inbound is shipped from location 9800000074. Based on this information, the company can evaluate better allocation of resources to better prepare for inbound shipped from this location.