Difference between revisions of "IS428 AY2019-20T2 Assign KANG HUI YUN"
Line 118: | Line 118: | ||
==== Tableau Desktop ==== | ==== Tableau Desktop ==== | ||
Upon importing the dataset to Tableau Desktop, we will make final adjustments to the dataset. | Upon importing the dataset to Tableau Desktop, we will make final adjustments to the dataset. | ||
− | + | <p>1. Firstly, arrange and set the fields according to the right format (i.e. Dimension or Measure, data type—String, Date, Boolean, etc).</p> | |
− | + | <p>2. Then, we will create a Group based on Question No to group different bunch of questions to their category (i.e. Communication, Service Delivery, Facilities & Equipment and Information Resources).</p> | |
− | + | <center>[[File:KANG HUI YUN-data TableauDesktop groupQuestionNoContextMenu.png|350px]] [[File:KANG HUI YUN-data TableauDesktop groupQuestionNo.png|250px]]</center> | |
+ | <p>3. Finally, we create hierarchies to better organise the related fields into logical subsets in our working area for better productivity due to the phenomenon known as ease of recall.</p> | ||
+ | <center>[[File:KANG HUI YUN-data TableauDesktop hierarchy.png|400px]] [[File:KANG HUI YUN-data TableauDesktop final.png|150px]]</center> | ||
== Interactive Data Visualisation == | == Interactive Data Visualisation == |
Revision as of 22:08, 15 March 2020
Overview
Background
In the interests of faculty, students and staff of Singapore Management University (SMU), an all-encompassing survey is conducted by SMU Libraries every 2 years to learn about their perception of the libraries (namely Li Ka Shing Library and Kwa Geok Choo Law Library), with particular focus on the importance and satisfaction level of different library-related services. This piece of information serves as a valuable input for the department to calibrate the standards of the facilities by identifying areas where users are underserved and those that exceed expectations but are relatively less important.
Objective & Tasks
After closure of the survey, a Library Survey Report is generated for consumption. However, this traditional method of analysis comprising huge number of tables and ineffective charts can be frustrating and difficult for users to grasp. Hence, the objective here is to make use of existing tools and technologies to develop an interactive visualisation of the survey data (Feb 2018), such that it is intuitive, easy to comprehend and telling at the same time. By cutting down on the report's "verbosity", the people in charge can save time deciphering trends and underlying patterns of the data with high degree of conciseness and accuracy.
The primary task is to reveal the level of services provided by the 2 SMU libraries as perceived by the following four groups: the undergraduates, postgraduates, faculty and staff. This mainly includes the concept of the Importance-Performance (or Importance-Satisfaction) rating as well as the Net Promoter Score (NPS).
Survey Data
About the Data
The data for the 2018 Library Survey Report consists of:
- 2639 records (rows)
- 89 fields (columns), which includes
- A new column for ResponseID (unique to each respondent)
- All other 88 columns related to the original survey questions
The survey is predominantly made up of importance and performance rating (26 such questions, represented by 78 fields in the dataset). The 78 fields are spread across 3 main categories: importance, performance and N/A (hence 26x3, giving us 78). Respondents who picked 'N/A' for the question need not select a rating for both importance and performance. Prior to these questions, there are 4 basic background and demographic questions that are all non-quantitative. Also, there are 3 questions on the frequency (of visits and access), 2 rating scales on overall satisfaction and likelihood of recommendation, and one textual input for comments and suggestions. For the most part, the survey questions are based on Likert scale (questions with suffixes between 1-27 except those with NA prefix; and out of the 27, 26 are the previously mentioned Importance-Performance rating questions). Worthy of mention is yet another rating scale—the Net Promoter Score (NPS), which, when calculated can tell us the overall (net) satisfaction/loyalty of library users.
Data Cleaning & Preparation
Before jumping into data visualisation, it is important to make sure the data is clean, rightfully organised and structured. This will facilitate the visual development using tools such as Tableau, allowing for categorisation and sorting. In the cleaning process, we shall engage in steps such as removal and/or creation of new fields, field renaming, and pivoting. Finally, we will piece up the bit-sized information into a single dataset via merging (e.g. join/union). Ultimately, we would like our data structure to be thin and tall (i.e. less columns, more rows) so we can analyse certain dimensions easily as we wish.
Initially, I have used Tableau Prep to clean the data. However, I quickly switched to using R (on RStudio) mainly because certain steps are tedious when performed in Tableau Prep—it requires a lot of manual work, especially repetitive clicking and entering of values. Writing a single R script is much cleaner, significantly faster and less prone to undesired changes which can be made through accidental interactions within the interface of Tableau Prep. Nonetheless, I have successfully created the same dataset using both methods besides Excel.
Excel
As mentioned previously, if the respondent selects N/A for a given question (which is represented as 1 in dataset under column NAxx), he/she will not be able to select an option for both Importance and Performance for the same question. Similarly, if N/A is not selected, it logically follows that he/she must select a choice for both Importance and Performance. However, there are records in the dataset that violate this rule. For example, the record where ResponseID is 765 has empty cells for both I02 and P02. Yet, NA2 is not filled with 1 when it should be.
To simplify the data cleaning process later, we will fix this issue by removing such records in Excel first.
Now we have successfully removed invalid records with regards to the questions. In the next phase, we will be able to remove the NAxx columns and focus on transforming other aspects of the data using R as it will be more efficient.
Tableau Prep Flow
Below shows the initial data cleaning process (flow) using Tableau Prep before switching to R.
R and RStudio
Step | Screenshot | Rationale & Description |
---|---|---|
Import Libraries | Import the libraries that are required to perform certain functions for data manipulation later on. | |
Import XLSX | Read in the Excel file that we have saved previously. | |
Remove Fields | First, we remove fields that are not of interest in the analysis. In this case, we remove Comment1 as sentiment analysis (via text mining) is not in our scope. Also, we can remove the 26 NA-prefixed fields as it is redundant (as we can refer back to Importance and Performance values to deduce whether user selected N/A). | |
Rename Field Names | We then rename the columns from the coded version to human-understandable words/phrases so that it is not confusing when working out the visualisation in Tableau. | |
Recode Field Values | Again, we recode the field values to human-understandable words/phrases to avoid confusion when working out the visualisation in Tableau. | |
Split Data | First, we have to split our data into smaller subsets with the interested fields to be pivoted. In our case, we wish to distinguish between whether a question-score pair belongs to the Importance or Performance factor, as well as the nature of the frequency question (i.e. whether the frequency is for library visits, campus visits or resource access). Hence, we will split the dataset into 3 smaller pieces. | |
Pivot Data | With the individual subsets, we then pivot the relevant columns for each subset to get the data structure we need. | |
Merge Subsets | Subsequently, we will merge the subsets together back into a single dataset. | |
Create New Field | Here, we will create a new field to generalise the respondents into a bigger group (i.e. Undergraduate, Postgraduate, Faculty, Staff and Others). For exchange students, we will group them along with the undergraduates. | |
Reorder Field | This step is not mandatory. However, it allows me to view/check the dataframe more efficiently when fields are logically grouped. | |
Remove Invalid Records | Now, we will find out invalid records and remove them from the dataset. Invalid records include those with empty or null values for the columns except Score. | |
Export Data | Finally, we export the R dataframe into a CSV! |
Tableau Desktop
Upon importing the dataset to Tableau Desktop, we will make final adjustments to the dataset.
1. Firstly, arrange and set the fields according to the right format (i.e. Dimension or Measure, data type—String, Date, Boolean, etc).
2. Then, we will create a Group based on Question No to group different bunch of questions to their category (i.e. Communication, Service Delivery, Facilities & Equipment and Information Resources).
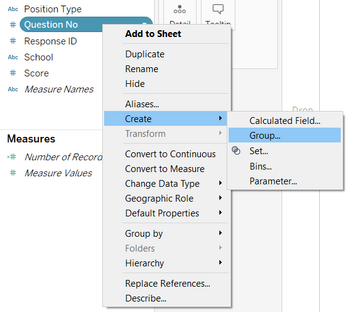
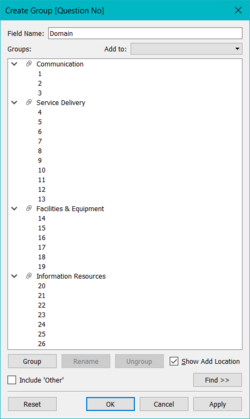
3. Finally, we create hierarchies to better organise the related fields into logical subsets in our working area for better productivity due to the phenomenon known as ease of recall.
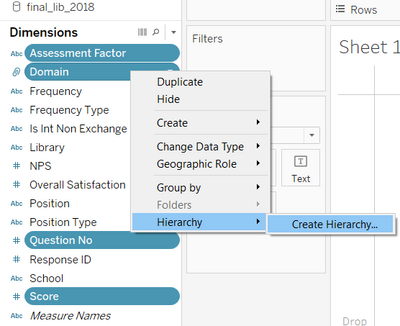
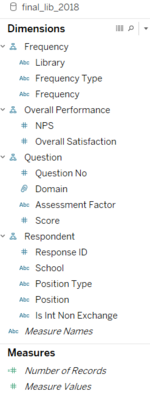